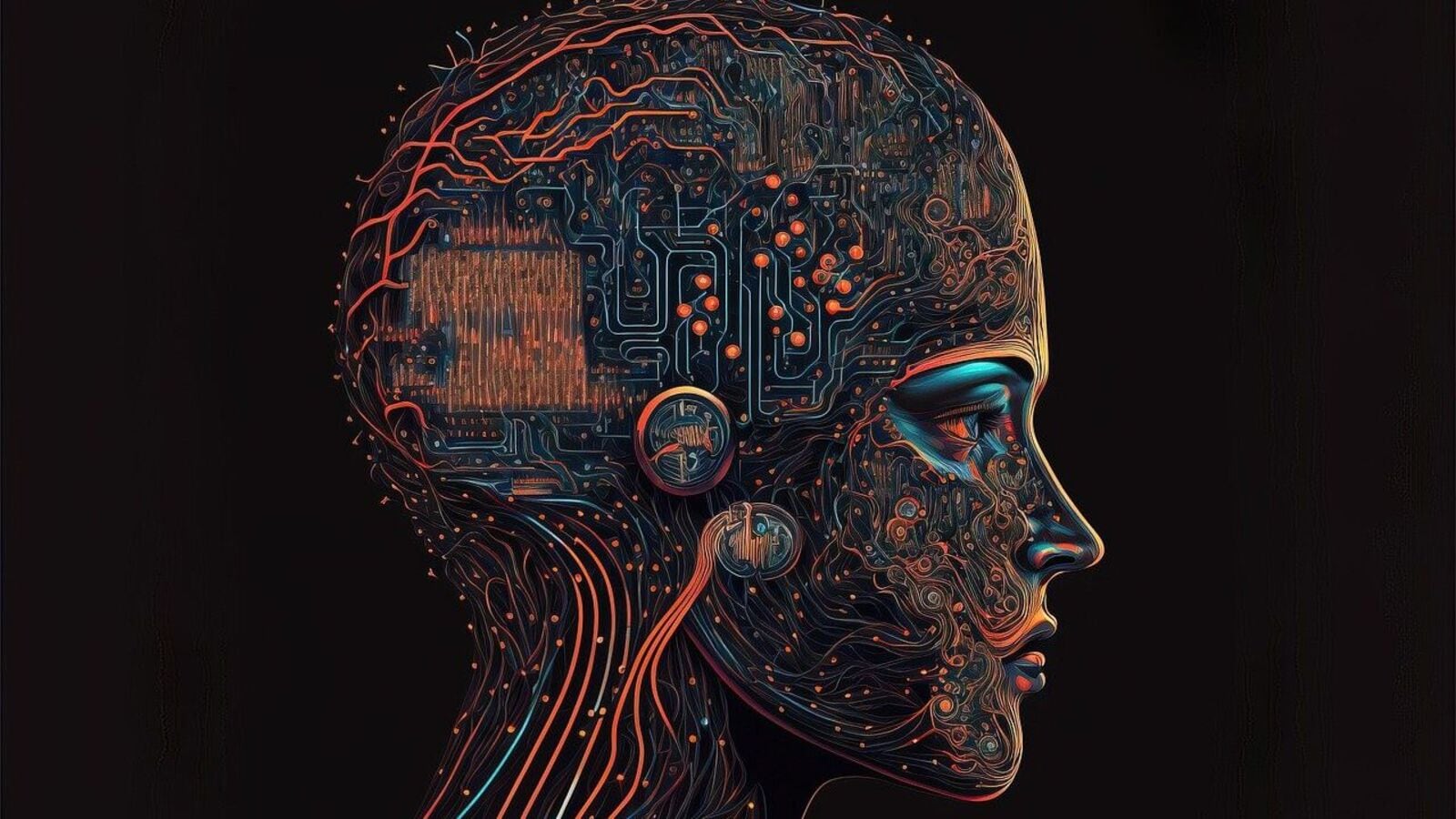
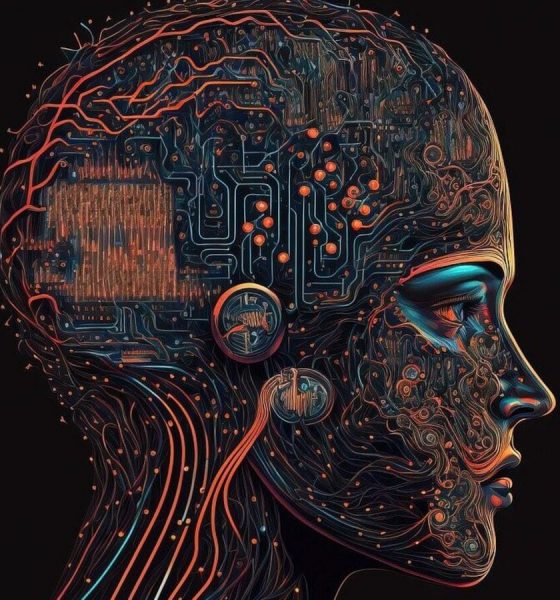
Metaverse
A short history of AI – Crypto News
The Dartmouth meeting did not mark the beginning of scientific inquiry into machines which could think like people. Alan Turing, for whom the Turing prize is named, wondered about it; so did John von Neumann, an inspiration to McCarthy. By 1956 there were already a number of approaches to the issue; historians think one of the reasons McCarthy coined the term artificial intelligence, later AI, for his project was that it was broad enough to encompass them all, keeping open the question of which might be best. Some researchers favoured systems based on combining facts about the world with axioms like those of geometry and symbolic logic so as to infer appropriate responses; others preferred building systems in which the probability of one thing depended on the constantly updated probabilities of many others.
View Full Image
The following decades saw much intellectual ferment and argument on the topic, but by the 1980s there was wide agreement on the way forward: “expert systems” which used symbolic logic to capture and apply the best of human know-how. The Japanese government, in particular, threw its weight behind the idea of such systems and the hardware they might need. But for the most part such systems proved too inflexible to cope with the messiness of the real world. By the late 1980s AI had fallen into disrepute, a byword for overpromising and underdelivering. Those researchers still in the field started to shun the term.
It was from one of those pockets of perseverance that today’s boom was born. As the rudiments of the way in which brain cells—a type of neuron—work were pieced together in the 1940s, computer scientists began to wonder if machines could be wired up the same way. In a biological brain there are connections between neurons which allow activity in one to trigger or suppress activity in another; what one neuron does depends on what the other neurons connected to it are doing. A first attempt to model this in the lab (by Marvin Minsky, a Dartmouth attendee) used hardware to model networks of neurons. Since then, layers of interconnected neurons have been simulated in software.
These artificial neural networks are not programmed using explicit rules; instead, they “learn” by being exposed to lots of examples. During this training the strength of the connections between the neurons (known as “weights”) are repeatedly adjusted so that, eventually, a given input produces an appropriate output. Minsky himself abandoned the idea, but others took it forward. By the early 1990s neural networks had been trained to do things like help sort the post by recognising handwritten numbers. Researchers thought adding more layers of neurons might allow more sophisticated achievements. But it also made the systems run much more slowly.
A new sort of computer hardware provided a way around the problem. Its potential was dramatically demonstrated in 2009, when researchers at Stanford University increased the speed at which a neural net could run 70-fold, using a gaming PC in their dorm room. This was possible because, as well as the “central processing unit” (cpu) found in all pcs, this one also had a “graphics processing unit” (gpu) to create game worlds on screen. And the gpu was designed in a way suited to running the neural-network code.
Coupling that hardware speed-up with more efficient training algorithms meant that networks with millions of connections could be trained in a reasonable time; neural networks could handle bigger inputs and, crucially, be given more layers. These “deeper” networks turned out to be far more capable.
The power of this new approach, which had come to be known as “deep learning”, became apparent in the ImageNet Challenge of 2012. Image-recognition systems competing in the challenge were provided with a database of more than a million labelled image files. For any given word, such as “dog” or “cat”, the database contained several hundred photos. Image-recognition systems would be trained, using these examples, to “map” input, in the form of images, onto output in the form of one-word descriptions. The systems were then challenged to produce such descriptions when fed previously unseen test images. In 2012 a team led by Geoff Hinton, then at the University of Toronto, used deep learning to achieve an accuracy of 85%. It was instantly recognised as a breakthrough.
By 2015 almost everyone in the image-recognition field was using deep learning, and the winning accuracy at the ImageNet Challenge had reached 96%—better than the average human score. Deep learning was also being applied to a host of other “problems…reserved for humans” which could be reduced to the mapping of one type of thing onto another: speech recognition (mapping sound to text), face-recognition (mapping faces to names) and translation.
In all these applications the huge amounts of data that could be accessed through the internet were vital to success; what was more, the number of people using the internet spoke to the possibility of large markets. And the bigger (ie, deeper) the networks were made, and the more training data they were given, the more their performance improved.
Deep learning was soon being deployed in all kinds of new products and services. Voice-driven devices such as Amazon’s Alexa appeared. Online transcription services became useful. Web browsers offered automatic translations. Saying such things were enabled by AI started to sound cool, rather than embarrassing, though it was also a bit redundant; nearly every technology referred to as AI then and now actually relies on deep learning under the bonnet.
In 2017 a qualitative change was added to the quantitative benefits being provided by more computing power and more data: a new way of arranging connections between neurons called the transformer. Transformers enable neural networks to keep track of patterns in their input, even if the elements of the pattern are far apart, in a way that allows them to bestow “attention” on particular features in the data.
Transformers gave networks a better grasp of context, which suited them to a technique called “self-supervised learning”. In essence, some words are randomly blanked out during training, and the model teaches itself to fill in the most likely candidate. Because the training data do not have to be labelled in advance, such models can be trained using billions of words of raw text taken from the internet.
Mind your language model
Transformer-based large language models (LLMs) began attracting wider attention in 2019, when a model called GPT-2 was released by OpenAI, a startup (GPT stands for generative pre-trained transformer). Such LLMs turned out to be capable of “emergent” behaviour for which they had not been explicitly trained. Soaking up huge amounts of language did not just make them surprisingly adept at linguistic tasks like summarisation or translation, but also at things—like simple arithmetic and the writing of software—which were implicit in the training data. Less happily it also meant they reproduced biases in the data fed to them, which meant many of the prevailing prejudices of human society emerged in their output.
In November 2022 a larger OpenAI model, GPT-3.5, was presented to the public in the form of a chatbot. Anyone with a web browser could enter a prompt and get a response. No consumer product has ever taken off quicker. Within weeks ChatGPT was generating everything from college essays to computer code. AI had made another great leap forward.
Where the first cohort of AI-powered products was based on recognition, this second one is based on generation. Deep-learning models such as Stable Diffusion and DALL-E, which also made their debuts around that time, used a technique called diffusion to turn text prompts into images. Other models can produce surprisingly realistic video, speech or music.
The leap is not just technological. Making things makes a difference. ChatGPT and rivals such as Gemini (from Google) and Claude (from Anthropic, founded by researchers previously at OpenAI) produce outputs from calculations just as other deep-learning systems do. But the fact that they respond to requests with novelties makes them feel very unlike software which recognises faces, takes dictation or translates menus. They really do seem to “use language” and “form abstractions”, just as McCarthy had hoped.
This series of briefs will look at how these models work, how much further their powers can grow, what new uses they will be put to, as well as what they will not, or should not, be used for.
© 2024, The Economist Newspaper Limited. All rights reserved. From The Economist, published under licence. The original content can be found on www.economist.com
-
Blockchain7 days ago
On-Chain Tokenization for Payments Professionals – Crypto News
-
Cryptocurrency1 week ago
Copper and P2P.org announce strategic collaboration to elevate institutional staking solutions – Crypto News
-
others1 week ago
Will Pi Network Price Crash or Rally After 212M Unlocks? – Crypto News
-
others1 week ago
Billionaire Barry Silbert Says This Is the Next Big Investment Theme for Crypto Assets – Crypto News
-
Cryptocurrency1 week ago
Breaking: Canary Capital Files For Staked Tron ETF – Crypto News
-
others1 week ago
Interoperability Protocol Asset Surges After a16z Acquires $55,000,000 Worth of the Project’s Native Asset – Crypto News
-
others6 days ago
GBP/USD retreats from YTD high past 1.34 on Fed turmoil – Crypto News
-
others5 days ago
Cantor Partners With Tether, SoftBank, Bitfinex For $3 Billion Bitcoin Bet – Crypto News
-
Cryptocurrency1 week ago
Manta Co-Founder ‘Targeted’ by Lazarus Group in Zoom Phishing Attempt – Crypto News
-
others1 week ago
Macro Guru Lyn Alden Predicts ‘Pretty Good Performance’ for Bitcoin Over the Coming Months – But There’s a Catch – Crypto News
-
Cryptocurrency1 week ago
Dow Jones Index Hangs On TSMC Earnings to Resist Slip. Will It Hold? – Crypto News
-
Technology1 week ago
Researchers Unveil 3D Tech That Lets Users ‘Touch’ Virtual Items – Crypto News
-
Business1 week ago
Coinbase Faces Renewed Legal Battle as Oregon Revives Old SEC Playbook – Crypto News
-
Technology1 week ago
Bitcoin Price Analysis: Two Weeks After Trump’s Tariffs, BTC Outperforms S&P 500 by 50% – Crypto News
-
Cryptocurrency1 week ago
Solana founders ‘returning to cypherpunk roots’: Colosseum’s Taylor – Crypto News
-
others1 week ago
Binance Reveals Major Update For Indian Users: Details – Crypto News
-
Blockchain1 week ago
Crypto, DeFi may widen wealth gap, destabilize finance: BIS report – Crypto News
-
Blockchain1 week ago
Cardano Whales Offload 180 Million ADA In 5 Days – Smart Profit-Taking? – Crypto News
-
Blockchain1 week ago
Altcoin unit bias ‘absolutely destroying’ crypto newbies — Samson Mow – Crypto News
-
Cryptocurrency1 week ago
Cardano Price Safeguards $0.600 Support, Upside Momentum Weakens – Crypto News
-
Cryptocurrency1 week ago
XRP bulls eye $2.60 – One move can trigger a major squeeze – Crypto News
-
Business1 week ago
Expert Says Solana Price To $2,000 Is Within Reach, Here’s How – Crypto News
-
Cryptocurrency7 days ago
Bybit CEO: Two-Thirds of Funds From $1.4B Lazarus Group Hack Still Traceable – Crypto News
-
others5 days ago
Australian Dollar receives support as private sector activity expands in April – Crypto News
-
others5 days ago
Tests 99.00 support after pulling back from nine-day EMA – Crypto News
-
Cryptocurrency5 days ago
Dogecoin ETF? 21Shares files as crypto market sees 12% rally – Crypto News
-
Blockchain1 week ago
Ethereum Price Stalls In Tight Range – Big Price Move Incoming? – Crypto News
-
Cryptocurrency1 week ago
Altcoins struggle while stablecoins shine: Is this the new normal? – Crypto News
-
Blockchain1 week ago
XRP to $50? Technical Analyst Lays Out the Roadmap – Crypto News
-
others1 week ago
SEC Greenlights New VanEck ‘Onchain Economy ETF’ That Holds Stocks Tied to the Digital Asset Sector – Crypto News
-
Blockchain1 week ago
Firing Jerome Powell will crash financial markets — Sen. Elizabeth Warren – Crypto News
-
Business1 week ago
Lorenzo Protocol (BANK) Price Rallies 150% After This Binance Announcement – Crypto News
-
Cryptocurrency1 week ago
Elizabeth Warren Warns Stock Market Will Crash If Trump Fires Powell, Will Crypto Market Crash Too? – Crypto News
-
Business7 days ago
XRP, Bitcoin, Ethereum Price Prediction: $100M Shorts Wiped as Crypto Market Bounces 2.89% – Crypto News
-
Technology6 days ago
Peter Schiff Predicts Gold Will Soar As Fed Cuts Rates, Will Bitcoin Price Follow? – Crypto News
-
Technology6 days ago
European Central Bank Claims Trump’s Crypto Push to Impact Europe Economy – Crypto News
-
Technology5 days ago
AWS, Microsoft Slow Down Data Center Deployments – Crypto News
-
Technology5 days ago
HashKey, Bosera partner to launch world’s first tokenized money market ETFs – Crypto News
-
Technology1 week ago
Can Shiba Inu Price Realistically Reach $0.0001? Elliot Wave Analysis Says Yes – Crypto News
-
Technology1 week ago
Special discounts for Amazon Prime members only! Up to 75% off on vacuum cleaners, water purifier, and more – Crypto News
-
Blockchain1 week ago
Ethereum Price Stalls In Tight Range – Big Price Move Incoming? – Crypto News
-
others1 week ago
GBP/USD remains on track to post weekly gains – Crypto News
-
Technology1 week ago
XRP Price Holds $2.08 Amid Derivatives Surge and Liquidation Risks – Crypto News
-
Technology1 week ago
OpenAI’s latest AI models are smarter, but they make things up more often. Here’s what we know – Crypto News
-
Blockchain1 week ago
Trump firing Powell would be a ‘very bad precedent to set’ — Pompliano – Crypto News
-
others1 week ago
Italy Trade Balance EU up to €-0.361B in February from previous €-0.635B – Crypto News
-
Blockchain1 week ago
Bitcoin Ready For $90K? ‘Next Big Move’ Could Come Next Week – Crypto News
-
Cryptocurrency1 week ago
Canary Capital Seeks SEC Approval for Tron ETF With Staking – Crypto News
-
others1 week ago
Peter Brandt Predicts 50% Crash For ‘Everyone’s Favorite’ XRP Price – Crypto News
-
others1 week ago
On-Chain Metrics Suggest Bitcoin (BTC) Could Be Approaching Early Bear Market Phase: Glassnode – Crypto News