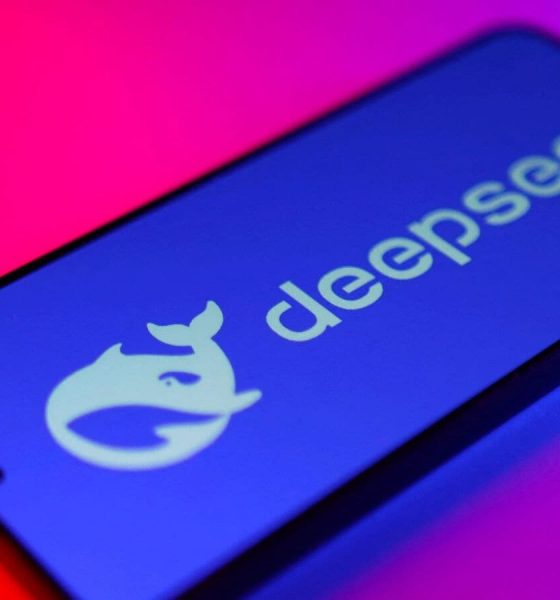
Metaverse
Forget DeepSeek. Large language models are getting cheaper still – Crypto News
As recently as 2022, just building a large language model (LLM) was a feat at the cutting edge of artificial-intelligence (AI) engineering. Three years on, experts are harder to impress. To really stand out in the crowded marketplace, an AI lab needs not just to build a high-quality model, but to build it cheaply.
In December a Chinese firm, DeepSeek, earned itself headlines for cutting the dollar cost of training a frontier model down from $61.6m (the cost of Llama 3.1, an LLM produced by Meta, a technology company) to just $6m. In a preprint posted online in February, researchers at Stanford University and the University of Washington claim to have gone several orders of magnitude better, training their s1 LLM for just $6. Phrased another way, DeepSeek took 2.7m hours of computer time to train; s1 took just under seven hours.
The figures are eye-popping, but the comparison is not exactly like-for-like. Where DeepSeek’s v3 chatbot was trained from scratch—accusations of data theft from OpenAI, an American competitor, and peers notwithstanding—s1 is instead “fine-tuned” on the pre-existing Qwen2.5 LLM, produced by Alibaba, China’s other top-tier AI lab. Before s1’s training began, in other words, the model could already write, ask questions, and produce code.
Piggybacking of this kind can lead to savings, but can’t cut costs down to single digits on its own. To do that, the American team had to break free of the dominant paradigm in AI research, wherein the amount of data and computing power available to train a language model is thought to improve its performance. They instead hypothesised that a smaller amount of data, of high enough quality, could do the job just as well. To test that proposition, they gathered a selection of 59,000 questions covering everything from standardised English tests to graduate-level problems in probability, with the intention of narrowing them down to the most effective training set possible.
To work out how to do that, the questions on their own aren’t enough. Answers are needed, too. So the team asked another AI model, Google’s Gemini, to tackle the questions using what is known as a reasoning approach, in which the model’s “thought process” is shared alongside the answer. That gave them three datasets to use to train s1: 59,000 questions; the accompanying answers; and the “chains of thought” used to connect the two.
They then threw almost all of it away. As s1 was based on Alibaba’s Qwen AI, anything that model could already solve was unnecessary. Anything poorly formatted was also tossed, as was anything that Google’s model had solved without needing to think too hard. If a given problem didn’t add to the overall diversity of the training set, it was out too. The end result was a streamlined 1,000 questions that the researchers proved could train a model just as high-performing as one trained on all 59,000—and for a fraction of the cost.
Such tricks abound. Like all reasoning models, s1 “thinks” before answering, working through the problem before announcing it has finished and presenting a final answer. But lots of reasoning models give better answers if they’re allowed to think for longer, an approach called “test-time compute”. And so the researchers hit upon the simplest possible approach to get the model to carry on reasoning: when it announces that it has finished thinking, just delete that message and add in the word “Wait” instead.
The tricks also work. Thinking four times as long allows the model to score over 20 percentage points higher on maths tests as well as scientific ones. Being forced to think for 16 times as long takes the model from being unable to earn a single mark on a hard maths exam to getting a score of 60%. Thinking harder is more expensive, of course, and the inference costs increase with each extra “wait”. But with training available so cheaply, the added expense may be worth it.
The researchers say their new model already beats OpenAI’s first effort in the space, September’s o1-preview, on measures of maths ability. The efficiency drive is the new frontier.
Curious about the world? To enjoy our mind-expanding science coverage, sign up to Simply Science, our weekly subscriber-only newsletter.
© 2025, The Economist Newspaper Limited. All rights reserved. From The Economist, published under licence. The original content can be found on www.economist.com
-
others1 week ago
Will Ethereum Price Rally to $3,200 as Wall Street Pivots from BTC to ETH – Crypto News
-
others5 days ago
Skies are clearing for Delta as stock soars 13% on earnings beat – Crypto News
-
others5 days ago
Skies are clearing for Delta as stock soars 13% on earnings beat – Crypto News
-
Cryptocurrency1 week ago
TON Foundation Confirms UAE Golden Visa Offer Is Not Official – Crypto News
-
others1 week ago
Company Owned by Billionaire Gold Miner May Be Seized by Russian Government for Allegedly Breaching Regulations: Report – Crypto News
-
Blockchain7 days ago
Insomnia Labs Debuts Stablecoin Credit Platform for Creators – Crypto News
-
Technology1 week ago
We’re Losing the Plot on AI in Universities – Crypto News
-
others1 week ago
Appropriate to have cautious gradual stance on easing – Crypto News
-
others6 days ago
EUR/GBP posts modest gain above 0.8600 ahead of German inflation data – Crypto News
-
Blockchain6 days ago
Ant Group Eyes USDC Integration Circle’s: Report – Crypto News
-
Cryptocurrency6 days ago
Bitcoin Breaks New Record at $111K, What’s Fueling the $120K Price Target? – Crypto News
-
Technology5 days ago
XRP Eyes $3 Breakout Amid Rising BlackRock ETF Speculation – Crypto News
-
Metaverse1 week ago
Are firms wasting their money on AI agents? – Crypto News
-
Metaverse1 week ago
Are firms wasting their money on AI agents? – Crypto News
-
Cryptocurrency1 week ago
Institutions Pile Up BTC But Price Doesn’t go up, Why? – Crypto News
-
others1 week ago
Bank Insider Admits to Nearly Decade-Long Scheme of Falsifying Loan Applications To Steal Funds: DOJ – Crypto News
-
Cryptocurrency1 week ago
This Week in Crypto Games: Planetside Dev’s ‘Reaper Actual’, What’s Next for ‘MapleStory Universe’ – Crypto News
-
Business1 week ago
Toncoin Price Drops 10% As UAE Authorities Call TON Golden Visa Offer Unofficial – Crypto News
-
Blockchain1 week ago
XRP Set To Shock The Crypto Market With 30% Share: Analyst – Crypto News
-
Cryptocurrency1 week ago
Coinbase hacker returns with $12.5 mln ETH buy: Will security concerns affect Ethereum? – Crypto News
-
others1 week ago
Is a Pi Network Crash Ahead As 272M Coins Unlock in July – Crypto News
-
Business1 week ago
Solana ETF Launch Delayed Amid Wait for SEC’s Crypto ETF Framework – Crypto News
-
Cryptocurrency1 week ago
On thinking ahead when markets get murky – Crypto News
-
Technology1 week ago
Solana Meme Coin PNUT Rallies 10% Amid Elon Musk’s Statement – Crypto News
-
Cryptocurrency1 week ago
Is ETH Finally Ready to Shoot For $3K? (Ethereum Price Analysis) – Crypto News
-
Cryptocurrency1 week ago
Tornado Cash Judge Won’t Let One Case Be Mentioned in Roman Storm’s Trial: Here’s Why – Crypto News
-
Blockchain7 days ago
XRP Rally Possible If Senate Web3 Crypto Summit Goes Well – Crypto News
-
others7 days ago
USD/CAD trades with positive bias below 1.3700; looks to FOMC minutes for fresh impetus – Crypto News
-
Blockchain7 days ago
Ethereum Bulls Roar — $3K Beckons After 5% Spike – Crypto News
-
Blockchain6 days ago
Kraken and Backed Expand Tokenized Equities to BNB Chain – Crypto News
-
others6 days ago
NovaEx Launches with a Security-First Crypto Trading Platform Offering Deep Liquidity and Institutional-Grade Infrastructure – Crypto News
-
Business6 days ago
Did Ripple Really Win XRP Lawsuit Despite $125M Fine? Lawyer Fires Back at CEO – Crypto News
-
Cryptocurrency6 days ago
XRP price forecast as coins surges 2.19% to $2.33 – Crypto News
-
others6 days ago
Anthony Scaramucci Says $180,000 Bitcoin Price Explosion Possible As BTC ‘Supremacy’ Creeps Up – Here’s His Timeline – Crypto News
-
Blockchain6 days ago
SUI Chart Pattern Confirmation Sets $3.89 Price Target – Crypto News
-
others5 days ago
EUR/GBP climbs as weak UK data fuels BoE rate cut speculation – Crypto News
-
Business5 days ago
PENGU Rallies Over 20% Amid Coinbase’s Pudgy Penguins PFP Frenzy – Crypto News
-
Cryptocurrency1 week ago
This Week in Crypto Games: Planetside Dev’s ‘Reaper Actual’, What’s Next for ‘MapleStory Universe’ – Crypto News
-
Blockchain1 week ago
Cardano (ADA) Turns Upward — Signs of a Recovery Emerge – Crypto News
-
Cryptocurrency1 week ago
Macroeconomics, Market Shifts, and Trading Speed Take Center Stage at B2MEET by B2PRIME – Crypto News
-
Blockchain1 week ago
UAE Golden Visa Is ‘Being Developed Independently‘ — TON Foundation – Crypto News
-
others1 week ago
Nasdaq-Listed Bit Digital Converts Entire Bitcoin Holdings To Ethereum Treasury – Crypto News
-
others1 week ago
Ethereum Continues Outperforming Institutional Capital Flows As Investors Pour $1,040,000,000 Into Crypto Products: CoinShares – Crypto News
-
Cryptocurrency1 week ago
Elon Musk announces his ‘America Party’ will embrace Bitcoin, criticizes Trump’s fiscal bill – Crypto News
-
others1 week ago
USD/CHF gains ground below 0.8000 ahead of US tariff deadline – Crypto News
-
Technology1 week ago
Huaweis AI lab denies that one of its Pangu models copied Alibabas Qwen – Crypto News
-
Blockchain1 week ago
EU Questions Robinhood About OpenAI and SpaceX Stock Tokens – Crypto News
-
Cryptocurrency1 week ago
XRP could rally higher on steady capital inflow; check forecast – Crypto News
-
Blockchain1 week ago
Vitalik Buterin Backs Copyleft Licensing for Fairer Crypto – Crypto News
-
Cryptocurrency1 week ago
Bulls In Control But Resistance Persists at $2.30. What Next? – Crypto News